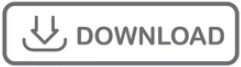
To get started we need to know what data we have. We will explore a real-world dataset, comprised of country-level socioeconomic data collected by GapMinder.
#Scatter plot relationships code#
The code for this project is available as a Jupyter Notebook on GitHub.
#Scatter plot relationships how to#
We will see how to create a default pairs plot for a rapid examination of our data and how to customize the visualization for deeper insights. In this article we will walk through getting up and running with pairs plots in Python using the seaborn visualization library. Pair plots are a great method to identify trends for follow-up analysis and, fortunately, are easily implemented in Python! A pairs plot allows us to see both distribution of single variables and relationships between two variables. While there are an almost overwhelming number of methods to use in EDA, one of the most effective starting tools is the pairs plot (also called a scatterplot matrix). EDA is the process of figuring out what the data can tell us and we use EDA to find patterns, relationships, or anomalies to inform our subsequent analysis. Once you’ve got yourself a nice cleaned dataset, the next step is Exploratory Data Analysis (EDA). How to quickly create a powerful exploratory data analysis visualization Also keep in mind that other factors may be involved in a cause-effect relationship.Visualizing Data with Pairs Plots in Python Of course this is not true!Īlways be careful what you infer from your statistical analyses. So, this must mean that the number of jars of strawberry jam sold in New York was causing an increase in the number of classical music CDs sold in Florida. The data was examined and was plottedįrom looking at the graph, it can be seen that there is a high positive correlation between these two sets of data. For the same time frame, the number of copies of a popular classical music CD sold in Florida was recorded. No correlation means that the data just doesn’t show if studying longer has any affect on Regents examination scores.Ĭheck out these graphs for visual interpretations of types of correlations:ĭuring the months of February and March, the weekly number of jars of strawberry jam sold at a local market in New York was recorded. If the plot on the graph is scattered in such a way that it does not approximate a line (it does not appear to rise or fall), there is no correlation between the sets of data. Under a negative correlation, the longer I study, the worse grade I would get on my Regents examination. If the slope of the line had been negative (falling from left to right), a negative correlation would exist since the slope of the line would have been negative. It all depends on the data being examined. There may be sets of data that show that there is NOT a positive correlation between hours studying and better Regents scores. Note: Just because this set of data showed a positive correlation does not mean that the relationship is positive for all sets of data concerning study time and Regents scores. This means that according to this set of data, the longer I study, the better grade I will get on my Regents examination. Since the slope of the line is positive, there is a positive correlation between the two sets of data. The data displayed on the graph resembles a line rising from left to right. Notice: Certain values may have more than one result, Remember when making a scatter plot, do NOT connect the dots. Given the data below, a scatter plot has been prepared to represent the data. Let’s decide if studying longer will affect Regents grades based upon a specific set of data.
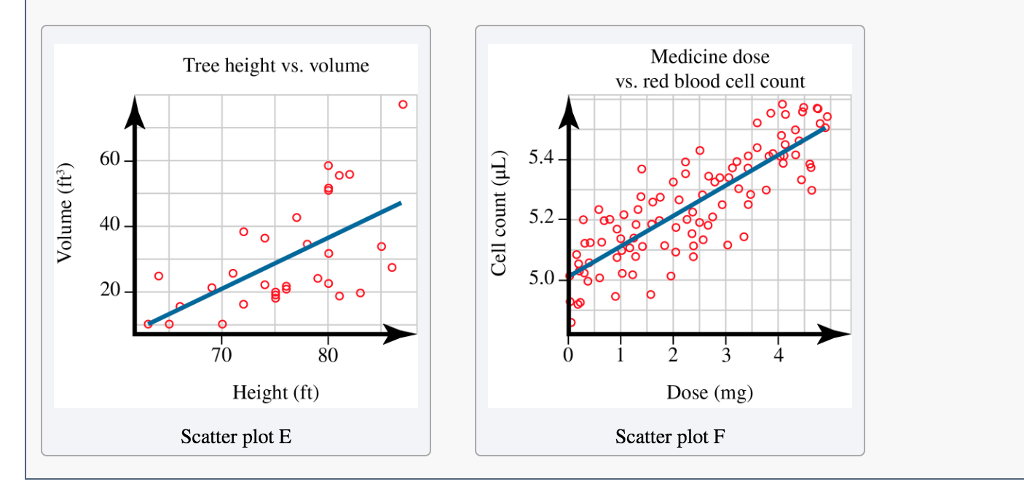
Scatter plots will often show at a glance whether a relationship exists between two sets of data. Statisticians and quality control technicians gather data to determine correlations (relationships) between such events.
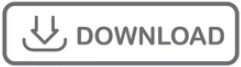